Efficiency & Effectiveness: Harnessing AI for Enhanced Productivity in Large Businesses
Razor-thin margins. Increasing cost pressures. Heightened customer expectations. How are large businesses able to deliver profitable growth in such operationally challenging times?
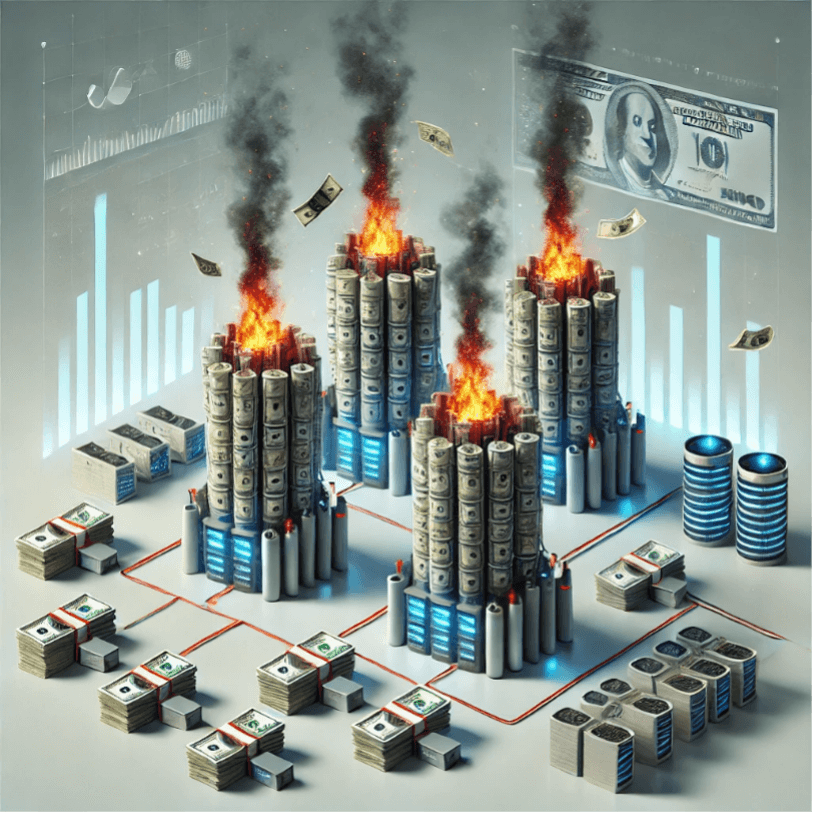
Most businesses have already delivered the easy wins and digitalised their offer. The new opportunity is looking deeper into core processes that underpin operations to unlock central productivity gains. The challenge? Siloed systems, with complex, “incompatible”, and fragmented data that rely on manual processes or hacked together micro-services.
The enemy of optimisation is a lack of interoperability
Interoperability is the ability of different systems, devices, or software to work together and exchange information seamlessly. Processes can only be fully optimised and insight fully gained if all relevant data and information can work together, with full automation again reliant on interoperability of data.
Most modern work processes rely on data that has been extracted, combined, and transformed between system’s which in turns rely on individuals with specific expertise. This requirement then creates key-person dependencies, another limiting factor to scale (efficiencies) and meaningful insights (applied ML and AI).
Faced with this system and data challenge, but with the need to deliver in year P&L savings, many businesses often fall into one of the following traps:
- Ignore these core processes and focus on easier-to-realise opportunities
- Streamline the process in department A, at the cost of increasing process and resource in department B.
- Invest capital in yet another expensive system
- Remove or simplify. Increasing efficiency but with a hidden cost of reducing effectiveness
The final scenario is the one we see most often, the typical “can’t have your cake and eat it” . An example of this is a large retailer that, when faced with a productivity challenge of saving headcount, chose to stop running a personalised, promotional email programme featuring the most relevant promotions for each customer. Then reverting to a multi-variant email approach.
This change stopped a number of manual processes that transferred and translated promotional and product data between systems, and reduced the number of compliance checks. Thus the cost saving was delivered and clearly visible in the corporate P&L. The downside was incremental sales from emails were impacted, as was the long term email engagement of customers. Both could be proven, however at the corporate level view of the world, these negative impacts were less initially apparent.
However reimagine that same retail marketing cost challenge scenario, one where you “can have your cake and eat it.”.Where the process for personalising the emails could not only be made more efficient — by automating many of the processes that sat between expensive, existing systems and automated compliance — but also more effective. For example, integrating additional data points that weren’t previously interoperable (i.e. easily compatible) into campaigns such as customers offline shopping behaviour, the type of imagery they most engaged with to increase click throughs, and factoring in current stock availability.
Not only would efficiency and costs saved increased, but effectiveness too, visible in sales, email engagement, and reduced customer complaints on products not being available.
With Northell, it’s possible
There’s no need to imagine. Our expert team use AI not just as a tool for automation, but as a transformative force that can fundamentally reshape how businesses operate. Our solutions work between your existing systems, addressing data and system silos to be able work together. This helps leverage your total data and knowledge base, in order to then automate processes and enhance the outputs.
A current example we are working on is with a global procurement provider. Their process of procuring materials is traditional and time consuming, reliant on years of colleague experience to navigate a complex supplier base with its myriad of systems, specs and data. All to ensure the optimal price for the customers specification and delivery requirements.
Our challenge in this example is to
- Codify business expertise, often hidden within a wealth of structured and unstructured data.
- Translate a myriad of different data sources, with different data formats, definitions and structures to create a holistic single data model. This single data model is then the basis for understanding how to source better including historical pricing, cost drivers, supplier performance and specialisms, component level procurement options etc.
- Using the data model to automate the end to end process by having a common language and data set.
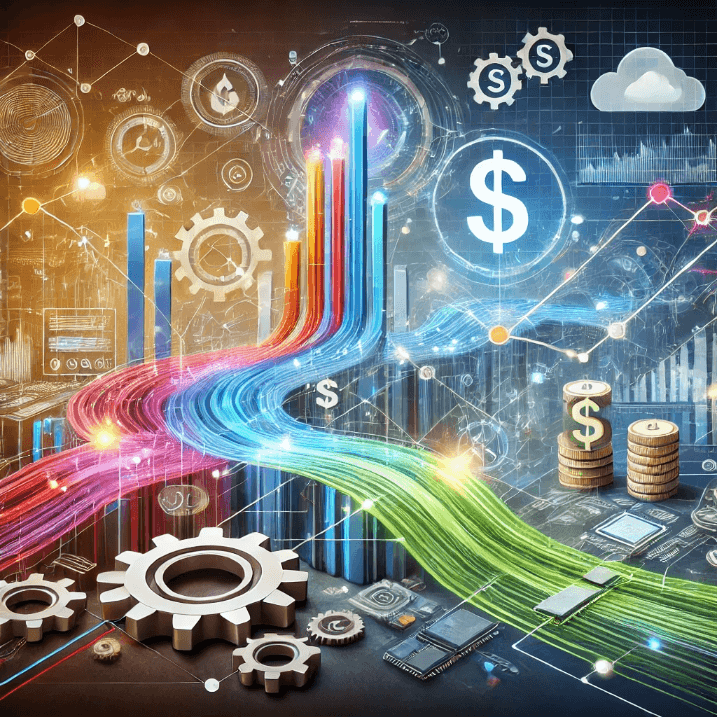
These three elements are then being combined to improve both the efficiency and effectiveness of the procurement process.
Get in touch to find out more or continue reading to learn more about the technology used to address the productivity challenges.
Ready to learn more about productivity challenges and the technology used to address them? Keep reading.
The Productivity Challenge in Large Enterprises
Large businesses often struggle with siloed data, fragmented processes, and the burden of managing vast amounts of unstructured information. These challenges can lead to inefficiencies, where valuable resources are wasted on manual tasks, and opportunities for strategic decision-making are missed due to the inability to harness the full potential of available data. Traditional automation solutions may improve efficiency to some extent, but they often fail to address the underlying issues of data integration and utilisation.
AI: Beyond Basic Automation
AI’s role in productivity is evolving beyond simple task automation. While early implementations of AI focused on automating repetitive, low-value tasks, the current wave of AI technologies is enabling businesses to optimise complex processes and make data-driven decisions in real time. This is achieved through the intelligent integration of various AI components, each playing a crucial role in transforming business operations.
The technologies behind Northell
Among the AI technologies that stand out in driving productivity improvements are Knowledge Graphs, Ontologies, Retrieval-Augmented Generation (RAG), and Compound AI Systems. All are integrated via a semantic layer that ensures both efficiency, and effectiveness in business activities.
The Role of Knowledge Graphs and Ontologies
At the core of this transformation are Knowledge Graphs and Ontologies. A Knowledge Graph is a structured representation of an organisation’s knowledge, connecting data points across different domains in a way that reflects their relationships and interdependencies. This interconnected data model enables businesses to break down silos and gain a holistic view of their operations.
Ontologies, on the other hand, provide a framework for understanding and categorising the data within these Knowledge Graphs. They define the relationships between concepts, allowing AI systems to interpret and reason with data in a more human-like manner. Together, Knowledge Graphs and Ontologies form the backbone of a semantic layer that makes enterprise data more accessible, interpretable, and actionable.
Retrieval-Augmented Generation (RAG): A New Paradigm in AI
RAG represents a significant advancement in AI’s ability to handle complex queries and generate accurate, contextually-relevant responses. Unlike traditional AI models that rely solely on pre-trained knowledge, RAG combines the power of retrieval mechanisms with generative models. This allows AI to access and integrate information from vast, up-to-date knowledge bases in real-time, providing answers that are not only correct, but also deeply informed by the latest available data.
For large businesses, this means that RAG can be used to automate decision-making processes that require extensive knowledge and context, such as customer service, product development, and strategic planning. By drawing on the rich, interconnected data stored in Knowledge Graphs, RAG-enabled systems can deliver insights and recommendations that are both accurate and timely.
Compound AI Systems: Integrating AI for Holistic Automation
Compound AI systems represent the integration of multiple AI technologies working in concert to address complex business challenges. These systems combine the strengths of different AI components—such as natural language processing, computer vision, machine learning, and RAG—to automate end-to-end processes across the enterprise.
For instance, in a large manufacturing company, a compound AI system might automate the entire supply chain process — from demand forecasting and inventory management, to production scheduling and logistics optimisation. By leveraging a semantic layer that integrates data from various sources, the AI system can not only streamline operations, but also adapt to changing conditions in real-time, ensuring that the business remains agile and responsive.
The Semantic Layer: Making Automation Effective
While automation improves efficiency by reducing manual intervention, the true power of AI lies in its ability to make processes more effective. This is where the semantic layer comes into play. By creating a unified, AI-ready data platform that integrates all business data —structured and unstructured —the semantic layer ensures that AI-driven processes are informed by the most comprehensive and relevant information available.
For example, in a global enterprise with multiple departments and data sources, the semantic layer can harmonise data from different systems, making it possible for AI to deliver consistent and accurate outputs across the organisation. This not only enhances the effectiveness of automated processes, but also ensures that business decisions are based on a complete and accurate picture of the enterprise.
Conclusion: The Future of Productivity is AI-Driven
As large businesses continue to navigate the complexities of a rapidly changing global market, the need for advanced AI solutions that go beyond basic automation becomes increasingly clear. By leveraging Knowledge Graphs, Ontologies, RAG, and Compound AI Systems, integrated through a semantic layer, enterprises can not only improve the efficiency of their operations but also enhance the effectiveness of their decision-making processes.
The future of productivity in large businesses lies in the intelligent application of AI—not just as a tool for automation, but as a strategic enabler of growth, innovation, and competitive advantage. As these technologies continue to evolve, they will undoubtedly play a central role in shaping the next generation of high-performing, data-driven enterprises.
To find out more about what we’re doing, or how we can help you get in touch.